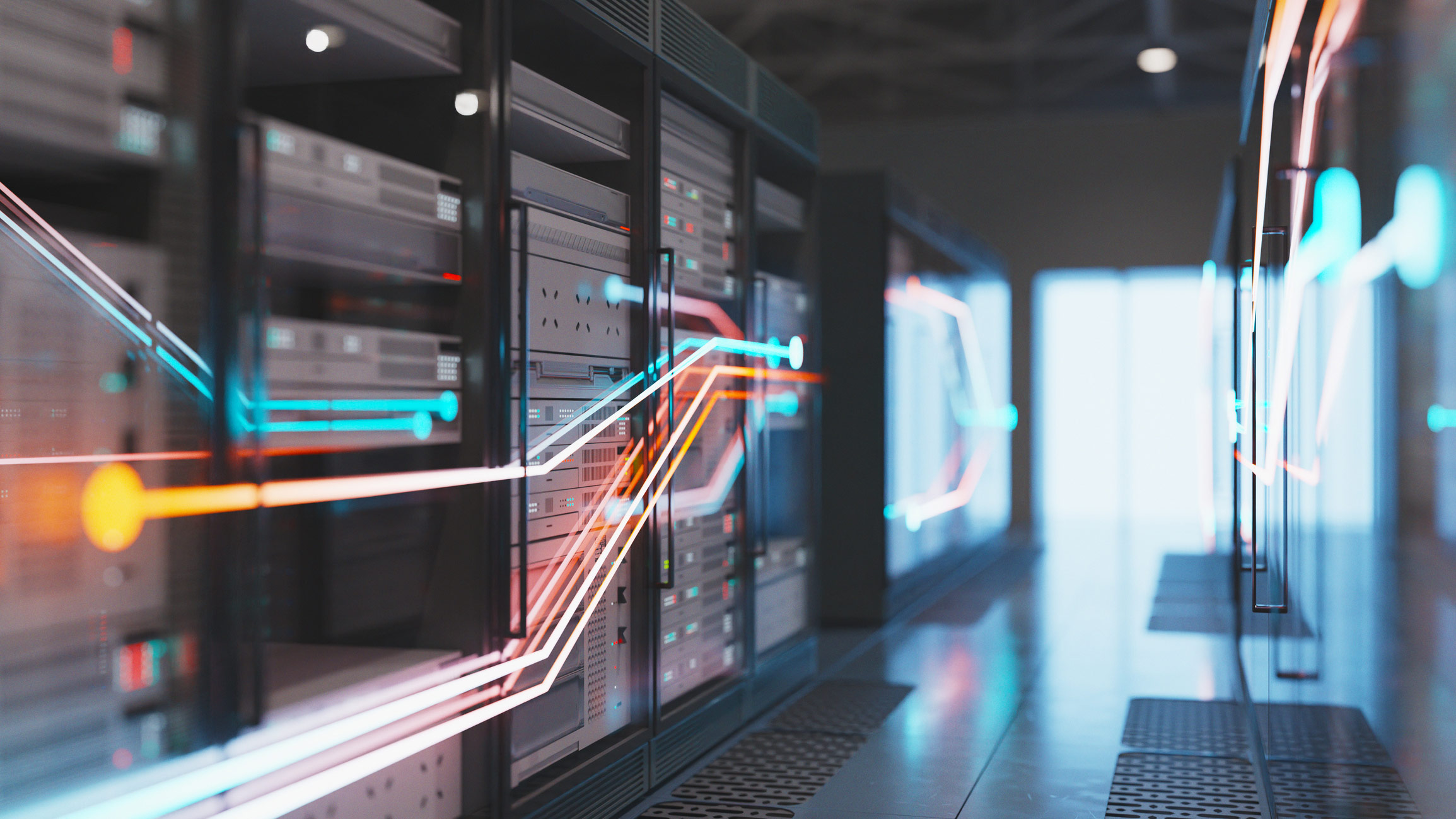
Data: Fueling the Future of Business
Over the last few decades, data & analytics has been associated with challenges like data volume, variety, data storage and real-time data processing but it also gives us the opportunity for better customer service, generating revenue growth, giving an edge over competitor and analytics on the motion. This is a review on data evolution journey, accessible data systems that comprise of tools and techniques to extract, transform, and load huge volume and variety of data. It also leverages the power of parallel processing to perform complicated transformations and analysis. Data analytics comes with a series of solution for business problems. The use of Data is becoming popular these days by the organizations to exceed their peers. In most of the industries, existing competitors and new entrants alike are using the strategies, resulting from the analyzed data to compete, innovate and achieve value.
We saw the era of oil in the global market in past decades, but this era would be an era of data, you saw topmost valued companies, 7 out of 10 are the data company. Data today is much more than the piece of information, it is fueling the future of most of the industries worldwide. Data has become essential for discovering success and has become a strategic asset
Data analytics is real-time processing of large and complex data sets which does not fit in the traditional relational database ecosystem. Data volume is so high that it is difficult to store and process this data for real-time analytics.
Second Global Technology Adoption Index study says, companies using Business data and Cloud technologies are experiencing up to 53% growth rates in revenue income than their counterparts. Let’s have more detail insights about data adoption journey of companies, the evolution of data and need of business data technologies.
Evolution of Data
Today we are generating approximately 3 quintillion byte of data. The primary source for huge data generation is smartphones, social media, IoT devices and online applications. Earlier we have candy phones which were used only for calling and sending text messages, now the smartphones are generating volumetric data. Today due to the immense technological advancements and our addiction to same, we all have become data-generating machines. We generate data in almost everything we do like, if you watch some YouTube video or read any blog, search, or buy any product online, use map/GPS to get directions, everything generates data, these are just the few examples, every day, every hour, every minute with the use of technology knowingly or unknowingly we generate huge volume of data.
Other than this now we are using Smart devices which really are IOT Devices, it is nothing but the physical devices which connect to the internet and make that device smart, now a days we have smart TVs, ACs, Watches, Cars and almost every appliance we use are IoT devices. These smart cars generate micro-level data using IOT devices like distance from obstruction, size of obstruction and others. So, you can imagine the data generated in 1 km of the drive. All these data are collected in an unstructured format which does not fit in the format that the relational database can handle or process for insight generation. A study says by 2021 around 1.7 MB data will be created every second by every human.
SMART city concept is one of the very good examples in today’s scenario Social CRM, Smart Parking which Monitors the availability of parking spaces in the city. Traffic Congestion – Monitoring of vehicles and pedestrian levels to optimize driving and walking routes and Smart Lighting intelligent and weather adaptive lighting in streetlights are the few examples of IoT deceive generated data. Other major source of data in today’s scenario is social media, social media data is one of the foremost factors for data evolution. Some of the examples are Facebook, YouTube, Instagram and Twitter, reviews, and comments online. Facebook pages you go around generates data, sharing of videos, likes on images/posts everything you do on social media generate data, when we order some product online that also generates data even some of our personal foots print like our address, name, age etc., these are few examples given over here that generate our history of purchase patterns.
Smart online buyers are very open and do not hesitate to write respective feedback and reviews on social media platforms. This high volumetric customer data that comes from enterprise applications, CRM, and other
social media platforms, can play a crucial role in understanding and unfolding the behavioral insight, sentiment analysis and paying attention to the voice of the customer.
Also, one very important part is that data is not in the structured format like a relational database, excel, on the other hand it’s huge. The volume, verity of data (structure, un-structure, and semi-structure) is the gest factor for the evolution of data.
What is Data Analytics?
Data analytics is a process of collecting, cleansing, combining, and consuming data with the goal of discovering useful insights and supporting decision-making. The collection of data set so large and complex that it becomes difficult to process using on-hand database system tools or traditional data processing applications, so we identify that our traditional old fashion system is not having the capability to process unstructured and large data sets, when traditional system was initially designed and invented, that time it was not intended for processing of large data sets. We didn’t did not anticipate this earlier that we must deal with such a huge volume of data. Now how do we consider data that needs special analytics?
Data gets detected and tracked from various sources which include transactional data from core business applications, social media, and information from machine-to-machine devices (IoT) or sensor data.
This creates huge volumes of data.
Tweets | 350K+ |
Facebook updates | 510K+ |
Instant Posts | 4.1K+ |
Google search | 4.2MM |
Emails | 210MM |
YouTube Videos’ watched | 3.47MM |
Data generated and stored in the last 2 years is more than the previous total data in history.
Data comes in various formats that may be structured like Excel file, CSV, numeric in the traditional database or the unstructured text documents, running text, feedback, video, audio, email, etc. this broadly can be categorized in 3 types – Structured, Semi-structured and unstructured.
The mining useful content/patterns and insight from large data set, you must ensure that data has been generated and processed should help for analytics. Authenticity of data needs to be check before processing and all the inconsistencies and anomalies need to be fixed. Multiple tools are available for data quality and data governance which enhance the data authenticity.
Data Analytics as an opportunity
Some of the popular examples of data analytics are – when you visit some online shopping site like Amazon or a social media site like YouTube for watching some videos, this site recommends some product or videos for you based on your buy or watch list, these recommendation systems provide real-time data analytics solution in few seconds.
One more example just pops on to my mind, Facebook uses data analytics to hide some of the sensitive image or video. They advise you before the subscription of that content. Earlier we are spending lots of money on data storage, few more problems like data were present in various formats like structured, semi-structured and non-structured. Now we understand how to store high volumes of data cost-effectively using commodity hardware or cloud infrastructure. It is more reliable and cost-effective. We found distributed file system as a solution and a better way to store the data. This saves a lot of money by using commodity hardware instead of dedicated data centers, these systems can handle all variety of data.
Data Analytics Case Study of Leading Public Sector Bank:
Problem Statement: A Leading Public Sector Bank in India wants to increase customer credit card usages. To Increase credit card usage bank wants to offer real-time messaging services that deliver location-based discount coupons by analyzing customer’s purchase patterns and tendencies by keeping track of when and where a customer purchased a particular item in real-time.
Solution: Bank has developed an Instant messaging system to deliver location-based discount coupons by analyzing customer’s purchase patterns and tendencies by using data analytics.
Other Business areas where Data Analytics Solution can prove handy and valuable.
Business Area | Business Problem | Data Analytics Solution |
---|---|---|
Customer Analytics | Who are my most Valuable Customers? Who can I cross sell my offerings to? Which of my Customers are likely to attrite? Which customers are likely to respond to campaigns? |
Lifetime Value Modelling Cross sell propensity Modelling Attrition Modelling Response Modelling |
Sales & Marketing | How can I optimize my Campaign ROI? How can I optimize cross sell offer management? How can I use Social Media Feedback? |
Uplift modelling Next Best Offer Analytics Social Media Analytics |
Loss Minimization |
How can I optimize my collection strategy and operations? | Collection’s scorecard |
(Collections) | How can I predict Asset Recovery behaviour? How can I track my collection efficacy? |
Asset Recovery optimization Collection’s dashboard |
Risk Mitigation and Fraud | How can I predict Customer Delinquency? How /can I identify likely Fraud before it happens? How to incorporate macro-economic factors in my risk decision system? |
Risk Score card Fraud Analytics Score card Stress testing |
Data Analytics Platform should exhibit the following features:
Simple. The simplest architecture is the best architecture. To apply this rule, Data strategy needs to be straight forward, data flow from source to data warehouse / repositories and all the report access through enterprise portal by user role and type. To reduce complexity, we strive to limit data duplication and advocate for a centralized uniform database platform, data assembly framework, and analytic platform.
User-Centric: Rather than focusing on the data or the technology required to collect, extract, ingest, clean, transform, and disseminate information, a data analytics architecture should begin with the end-users problem statement and their requirements.
Adaptable: In a Data analytics architecture, data flows like water from source systems to centralized data repositories which hold small and sharp data and provide more context for analytics. The purpose of the architecture is to manage that flow by creating a series of interconnected data pipelines that serve various user needs.
Automated. Data analytics should be an adaptable architecture in which data flows continuously, and the process and platform should automate everything. This should profile and time tagging of data as it is ingested and map it to existing data sets and repositories, a process called metadata injection, a key function of data catalogs. In a real-time environment, it must detect anomalies and notify the appropriate individuals or trigger alerts in operational dashboards.
Flexible. This architecture is flexible enough to support a multiplicity of business needs and follow open bus architecture.
Collaborative. Data analytics gives a collaborative environment to users. Unlike the past where the agency assembled everything. The Data analytics architecture splits the responsibility for acquiring and transforming data between business users across the departments. The implementation agency still does the heavy lifting of ingesting data from source systems and creating reusable data blocks. But from there, Department users take over if they have the skills, desire, and need.
Governed. A Data analytics architecture defines access points for each type of user to meet their information requirements which define the access points for four classes of department users: data consumers, data explorers, data analysts and data scientists. For instance, data scientists need to be given access to raw data in the data catalog.
Elastic. Elastic architecture that adapts to changing data processing requirements on demand. We should obtain on-demand scalability. Elastic architectures free administrators from having to calibrate capacity exactly, throttle usage if necessary, and overbuy hardware incessantly.
Secure. A data platform architecture is a freedom fortress—that is, it provides authorized users ready access to data while keeping unauthorized users & intruders at bay. It also complies with security guidelines, compliances, regulations, and other guidelines as well as policies.
Resilient. The data architecture shall be resilient, with high availability, disaster recovery, and backup/restore capabilities. This is especially true in a data architecture that often runs on huge server farms in the cloud where outages are commonplace.
Data helps the organizations to build new opportunities and entirely new categories of corporations that can analyse and consolidate a huge volume of data. These companies have ample information about the products and services, consumer choices and sentiments that can be captured and analysed.
Data analytics techniques can facilitate enterprises that offer comprehensive powerful and actionable insights into 360° customer view, customer and product classification, and sentiment analysis, Sales forecast. Analytical and statistical modelling allows an organization to forecast all portfolios and probable losses.
With limited understanding of data management and a lack of data-centric culture being the barriers, companies should rethink and adopt the data analytics services and understand the power of data. They need to incorporate cutting-edge technologies to get interesting data and figure out ways to use data in the data analytics journey.